Affect and Generative AI gives nice alternatives, however we additionally must handle danger
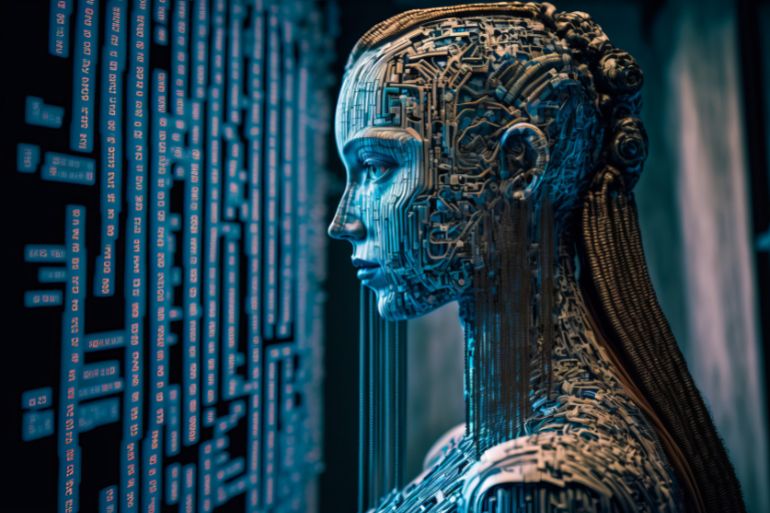
[ad_1]
Within the remaining week of March 2023, the Way forward for Life Institute made headlines with its open letter, signed by a few of the largest names in tech, calling on all synthetic intelligence (AI) labs to “instantly pause the coaching of AI techniques extra highly effective than GPT-4”.
It cited the necessity to enable security analysis and coverage to meet up with the “profound dangers to society and humanity” created by the fast development in AI capabilities.
Within the two months since, we’ve seen commentary from all sides in regards to the runaway progress of the AI Arms Race and what must be finished about it.
Sundar Pichai, CEO of Google and Alphabet, has lately mentioned that “constructing AI accountability is the one race that basically issues”, a mere few months after declaring a ‘code pink’ in response to the success of Open AI’s ChatGPT.
Governments are additionally on discover, with Members of the European Parliament having reached settlement on the EU’s flagship AI Act, and the US authorities investing US$140m into pursuing AI developments which are “moral, reliable, accountable and serve the general public good”.
The important thing query stays: how ought to we be eager about balancing the hazards in opposition to the alternatives arising from the mainstreaming of (generative) AI?
What’s AI?
AI is a sequence of components – together with sensors, information, algorithms and actuators, working in many alternative methods and with completely different functions. AI can also be a sociotechnical thought – a technical software making an attempt to automate sure features, however at all times based mostly in maths. Generative AI is only one type of AI.
The case for a brand new paradigm of AI danger evaluation
I lately spoke with Dr Kobi Leins, a worldwide professional in AI, worldwide regulation and governance, about how we should always conceptualise this delicate steadiness.
Dr Leins confused the necessity for rising the depth of our risk-analysis lens and actively contemplating the long-term, interconnected societal dangers of AI-related hurt, in addition to embracing potential advantages. She highlighted not solely the hazards of prioritising pace over security, but additionally urged a cautious method to searching for methods to make use of the applied sciences, somewhat than beginning with the enterprise issues and utilizing the toolbox of applied sciences accessible. Some instruments are cheaper and fewer dangerous, and should remedy the issue with out the (just about) rocket-fuelled resolution.
So what does this appear like?
Identified unknowns vs unknown unknowns
It’s essential to do not forget that the world has seen this magnitude of danger earlier than. Echoing a quote reputed to be by Mark Twain, Dr Leins informed me that “historical past by no means repeats itself, but it surely does usually rhyme.”
Many comparable examples of scientific failures inflicting immense hurt exist, the place advantages might have been gained and dangers averted. One such cautionary story lies in Thomas Midgley Jnr’s invention of chlorofluorocarbons and leaded gasoline – two of historical past’s most damaging technological improvements.
As Stephen Johnson’s account within the NY Instances highlights, Midgley’s innovations revolutionised the fields of refrigeration and car effectivity respectively and had been lauded as a few of the best developments of the early twentieth century.
Nonetheless, the passing of the following 50 years and the event of recent measurement expertise revealed that they had been to have disastrous results on the long-term way forward for our planet – particularly, inflicting the opening within the ozone layer and widespread lead poisoning. One other well-known instance is Einstein, who died having contributed to making a software that was used to hurt so many.
The lesson right here is obvious. Scientific developments that appear like nice concepts on the time and are fixing very actual issues can prove to create much more damaging outcomes in the long run. We already know that generative AI creates vital carbon emissions and makes use of vital quantities of water, and that broader societal points resembling misinformation and disinformation are trigger for concern.
The catch is that, as was the case with chlorofluorocarbons, the long-term harms of AI, together with generative AI, will very seemingly solely be absolutely understood over time, and alongside different points, resembling privateness, cybersecurity, human rights compliance and danger administration.
The case for extending the depth of our lens
Whereas we will’t but predict with any accuracy the longer term technological developments which may unearth the harms we’re creating now, Dr Leins emphasised that we should always nonetheless be considerably extending our timeframe, and breadth of imaginative and prescient, for danger evaluation.
She highlighted the necessity for a danger framing method centered on ‘what can go flawed’, as she discusses briefly in this episode of the AI Australia Podcast, and means that the most secure threshold needs to be disproving hurt.
We mentioned three areas wherein administrators and decision-makers in tech firms coping with generative AI needs to be eager about their method to danger administration.
- Contemplating longer timelines and use circumstances affecting minoritised teams
Dr Leins contends that we’re at the moment seeing very siloed analyses of danger in business contexts, in that decision-makers inside tech firms or startups usually solely take into account danger because it applies to their product or their designated utility of it, or the influence on individuals who appear like them or have the identical quantity of information and energy.
As an alternative, firms must do not forget that generative AI instruments don’t function in isolation, and take into account the externalities created by such instruments when used together with different techniques. What’s going to occur when the system is used for an unintended utility (as a result of this will occur), and the way does the entire system match collectively? How do these techniques influence the already minoritised or weak, even with moral and consultant information units?
Vital work is already being finished by governments and policymakers globally on this house, together with within the growth of the ISO/IEC 42001 customary for AI, designed to make sure implementation of round processes of building, implementing, sustaining and regularly enhancing AI after a software has been constructed.
Whereas top-down governance will play an enormous function in the way in which ahead, the onus additionally sits with firms to be a lot better at contemplating and mitigating these dangers themselves.
Outsourcing danger to 3rd events or automated techniques won’t solely not be an choice, however it might trigger additional dangers that companies will not be considering but past third celebration danger, provide chain dangers and SaaS dangers.
- Excited about the precise options
Corporations must also be asking themselves what their precise targets are and what the precise instruments to repair that downside actually appear like, after which choose the choice that carries the least danger. Dr Leins instructed that AI isn’t the answer to each downside, and subsequently shouldn’t at all times
be used as the place to begin for product growth. Leaders needs to be extra discerning in contemplating whether or not it’s price taking up the dangers within the circumstances.
Begin from an issue assertion, have a look at the toolbox of applied sciences, and resolve from there, somewhat than making an attempt to assign applied sciences to an issue.
There’s a number of hype in the intervening time, however there may also be more and more obvious danger. Come fast to undertake generative AI have already stopped utilizing it – as a result of it didn’t work, as a result of it absorbed mental property, or as a result of it utterly fabricated content material indiscernible from truth.
- Cultural change inside organisations
Corporations are sometimes run by generalists, with enter from specialists. Dr Leins informed me that there’s at the moment a cultural piece lacking that should change – when the AI and ethics specialists ring the alarm bells, the generalists must cease and pay attention. Variety on groups and having completely different views can also be crucial, and though many elements of AI are at the moment already ruled, gaps stay.
We will take a lesson right here from the Japanese manufacturing upkeep precept referred to as ‘andon’, the place each member of the meeting line is seen as an professional of their discipline and has the facility to full on the ‘andon’ wire to cease the road in the event that they spot one thing they understand to be a menace to manufacturing high quality.
If somebody wherever in a enterprise identifies a problem with an AI software or system, administration ought to cease, pay attention, and take it very critically. A tradition of security is essential.
Closing ideas
Founders and startups needs to be listening out for alternatives with AI and automation, but additionally maintain a wholesome cynicism about a few of the ‘magical options’ being touted. This contains boards establishing a danger urge for food that’s mirrored in inside frameworks, insurance policies and danger administration, but additionally in a tradition of curiosity and humility to flag issues and danger.
We’re not saying it ought to all be doom and gloom, as a result of there’s undoubtedly lots to be enthusiastic about within the AI house.
Nonetheless, we’re eager to see the dialog proceed to evolve to make sure we don’t repeat the errors of the previous, and that any new instruments assist the values of environmentally sustainable and equitable outcomes.
[ad_2]